基于深度学习的侧信道攻击指北
本文是关于侧信道攻击入门的指北,不适合未满8岁的人士食用,未成年人请在成年人的陪同下浏览。本人对指北内容概不负责,如有眩晕、呕吐等不适情况请立刻关闭该网页并及时就医。
论文(持续补充)
这里是本人看过(下载过)的论文,会持续补充。
# | 题目 | 站内链接 | 论文链接 | 类型 |
---|---|---|---|---|
0 | Study of Deep Learning Techniques for Side-Channel Analysis and Introduction to ASCAD Database | click | link | 入门必看 |
1 | One Network to rule them all. An autoencoder approach to encode datasets | click | link | 特征提取 |
2 | Multilabel Deep Learning-Based Side-Channel Attack | click | link | 多标签学习 |
3 | Deep Learning based Side-Channel Attack: a New Profiling Methodology based on Multi-Label Classification | click | link | 多标签学习 |
4 | CDAE: Towards Empowering Denoising in Side-Channel Analysis | click | link | 降噪 |
5 | On the Performance of Convolutional Neural Networks for Side-channel Analysis | click | link | 网络架构 |
6 | To Overfit, Or Not to Overfit: Improving the Performance of Deep Learning-based SCA | click | link | 炼丹指导 |
7 | The Best of Two Worlds: Deep Learning-assisted Template Attack | click | link | 特征提取方法 |
8 | Pay Attention to Raw Traces: A Deep Learning Architecture for End-to-End Profiling Attacks | link | 端到端侧信道攻击 | |
9 | Cross-Device Profiled Side-Channel Attack with Unsupervised Domain Adaptation | link | 跨设备侧信道攻击 | |
10 | Methodology for Efficient CNN Architectures in Profiling Attacks | link | 网络架构 | |
11 | It’s a Kind of Magic: A Novel Conditional GAN Framework for Efficient Profiling Side-channel Analysis | link | 特征提取 | |
12 | I Know What Your Layers Did: Layer-wise Explainability of Deep Learning Side-channel Analysis | link | ||
13 | Revisiting a Methodology for Efficient CNN Architectures in Profiling Attacks | click | link | 练手用 |
14 | Don’t Learn What You Already Know Scheme-Aware Modeling for Profiling Side-Channel Analysis against Masking | link | 没看懂 | |
15 | Exploring Feature Selection Scenarios for Deep Learning-based Side-channel Analysis | click | link | 特征工程 |
16 | Side-channel analysis against ANSSI’s protected AES implementation on ARM: end-to-end attacks with multi-task learning | link | 多任务学习 | |
17 | Multi-Leak Deep-Learning Side-Channel Analysis | link | 多点泄露攻击 | |
18 | Deep Learning Side-Channel Collision Attack | link | 碰撞攻击(新 | |
19 | Remove Some Noise: On Pre-processing of Side-channel Measurements with Autoencoders | click | link | 降噪 |
20 | THE CIRCLE OF DL-SCA | — | link | 吴立超博士毕业论文 |
21 | I Choose You: Automated Hyperparameter Tuning for Deep Learning-based Side-channel Analysis | link | 超参数自动调整 | |
22 | Hiding in Plain Sight: Non-profiling Deep Learning-based Side-channel Analysis with Plaintext/Ciphertext | link | 非建模侧信道攻击 | |
23 | Breaking Cryptographic Implementations Using Deep Learning Techniques | |||
24 | SoK: Deep Learning-based Physical Side-channel Analysis | link | 综述 | |
25 | Fake it till you make it: Data Augmentation using Generative Adversarial Networks for all the crypto you need on small devices | link | 数据增强 | |
26 | ||||
27 | The Need for Speed: A Fast Guessing Entropy Calculation for Deep Learning-Based SCA | link | 训练中callback | |
28 | Ranking Loss: Maximizing the Success Rate in Deep Learning Side-Channel Analysis | link | 损失函数 | |
29 | Label Correlation in Deep Learning-Based Side-Channel Analysis | link | 标签相关性 | |
30 | Breaking Free: Leakage Model-free Deep Learning-based Side-channel Analysis | link | 泄露模型 | |
31 | Pay Attention to Raw Traces: A Deep LearningArchitecture for End-to-End Profiling Attacks | link | 攻击原始能量迹 | |
32 | Deep Learning Side-Channel Analysis on Large-Scale Traces A Case Study on a Polymorphic AES | link | 攻击大规模能量迹 | |
33 | Non-Profiled Deep Learning-based Side-Channel attacks with Sensitivity Analysis | link | 敏感值分析 | |
34 | EstraNet: An Efficient Shift-Invariant Transformer Network for Side-Channel Analysis | link | Trasformer 侧信道 | |
35 | A Comprehensive Study of Deep Learning for Side-Channel Analysis | — | link | NLL |
36 | Leakage Certification Revisited: Bounding Model Errors in Side-Channel Security Evaluations | link | 感知信息 | |
37 | Perceived Information Revisited New Metrics to Evaluate Success Rate of Side-Channel Attacks | link | 感知信息pro | |
38 | Perceived Information Revisited II Information-Theoretical Analysis of Deep-Learning Based Side-Channel Attacks | link | 感知信息pro max | |
39 | AutoPOI: automated points of interest selection for side-channel analysis | link | 自动提取兴趣点 | |
40 | Weakly Profiling Side-channel Analysis | link | 新建模方法 | |
41 | Plaintext: A Missing Feature for Enhancing the Power of Deep Learning in Side-Channel Analysis? Breaking multiple layers of side-channel countermeasures | link | 提升针对掩码的破坏性 | |
42 | Strength in Numbers: Improving Generalization with Ensembles in Machine Learning-based Profiled Side-channel Analysis | link | 集成学习 | |
43 | Give Me 5 Minutes Attacking ASCAD with a Single Side-Channel Trace | link | SCALib的广告 | |
44 | Mind the Portability: A Warriors Guide through Realistic Profiled Side-channel Analysis | link | 跨设备侧信道攻击 | |
45 | Ablation Analysis for Multi-Device Deep Learning-Based Physical Side-Channel Analysis | link | 跨设备侧信道攻击 | |
46 | Adaptive Chosen-Plaintext Deep Learning-based Side-Channel Analysis | link | ||
47 | How Far Can We Reach? Breaking RSM-Masked AES-128 Implementation | link | RSM掩码攻击 | |
48 | Sneaking up the Ranks: Partial Key Exposure Attacks on Rank-Based Schemes | link | rank | |
49 | Manifold Learning Side-Channel Attacks against Masked Cryptographic Implementations | link | 流形学习侧信道 | |
50 | Single-trace side-channel attacks on MAYO exploiting leaky modular multiplication | link | 后量子侧信道攻击 | |
51 | One Solves All: Exploring ChatGPT’s Capabilities for Fully Automated Simple Power Analysis on Cryptosystems | link | GPT-SPA | |
52 | Can KANs Do It? Toward Interpretable Deep Learning-based Side-channel Analysis | link | KAN-SCA | |
53 | Creating from Noise: Trace Generations Using Diffusion Model for Side-Channel Attack | link | 扩散模型-sca | |
54 | Leakage Model-flexible Deep Learning-based Side-channel Analysis | link | 多任务多标签侧信道攻击 | |
55 | Scoring the predictions: a way to improve profiling side-channel attacks | link | 排名优化 | |
56 | Regularizers to the rescue: fighting overfitting in deep learning-based side-channel analysis | link | 过拟合优化 | |
58 | CL-SCA: Leveraging Contrastive Learning for Profiled Side-Channel Analysis | link | 对比学习 | |
60 | Autoencoder-enabled model portability for reducing hyperparameter tuning efforts in side-channel analysis | link | 超参数调整优化策略 | |
61 | Diffuse Some Noise: Diffusion Models for Measurement Noise Removal in Side-channel Analysis | link | 扩散模型去噪 | |
62 | Blind-Folded: Simple Power Analysis Attacks using Data with a Single Trace and no Training | link | 单条能量迹、SPA | |
63 | Side-Channel Attacks Based on Multi-Loss Regularized Denoising AutoEncoder | [link](Side-Channel Attacks Based on Multi-Loss Regularized Denoising AutoEncoder) | 去噪编码器 | |
64 | HierNet: A Hierarchical Deep Learning Model for SCA on Long Traces | link | 原始能量迹攻击 | |
65 | On the Instability of Softmax Attention-Based Deep Learning Models in Side-Channel Analysis | link | 注意力 | |
66 | Conditional Variational AutoEncoder based on Stochastic Attacks | link | 条件变分自编码器 | |
67 | Beyond the Last Layer: Deep Feature Loss Functions in Side-channel Analysis | link | 损失函数 | |
68 | OccPoIs: Points of Interest based on Neural Network’s Key Recovery in Side-Channel Analysis through Occlusion | link | ||
69 | Peek into the Black-Box: Interpretable Neural Network using SAT Equations in Side-Channel Analysis | link | 可解释性研究 | |
70 | An End-to-end Plaintext-based Side-channel Collision Attack without Trace Segmentation | link | 碰撞攻击 | |
71 | SCA-CGAN:A New Side-Channel Attack Method for Imbalanced Small Samples | link | 小样本侧信道攻击 | |
72 | The Need for MORE: Unsupervised Side-channel Analysis with Single Network Training and Multi-output Regression | link | 无监督侧信道攻击 | |
73 | Tipping the Balance: Imbalanced Classes in Deep Learning Side-channel Analysis | link | 标签不平衡研究 | |
74 | The Side-channel Metrics Cheat Sheet | link | 好文! | |
75 | No (good) loss no gain: systematic evaluation of loss functions in deep learning-based side-channel analysis | link | 损失函数设计 | |
76 | It’s a Kind of Magic: A Novel Conditional GAN Framework for Efficient Profiling Side-channel Analysis | link | GAN | |
77 | Efficient Exploitation of Noise Leakage for Template Attack | link | 模板攻击 | |
78 | Encoding Power Traces as Images for Efficient Side-Channel Analysis | link | 图像侧信道 | |
79 |
Mathematical Foundations for Side-Channel Analysis of Cryptographic Systems 侧信道攻击的数学基础,但是学校没springer的Institutional subscriptions!!!
指北
这部分解释侧信道攻击以及本人研究方向的内容。
什么是侧信道攻击?
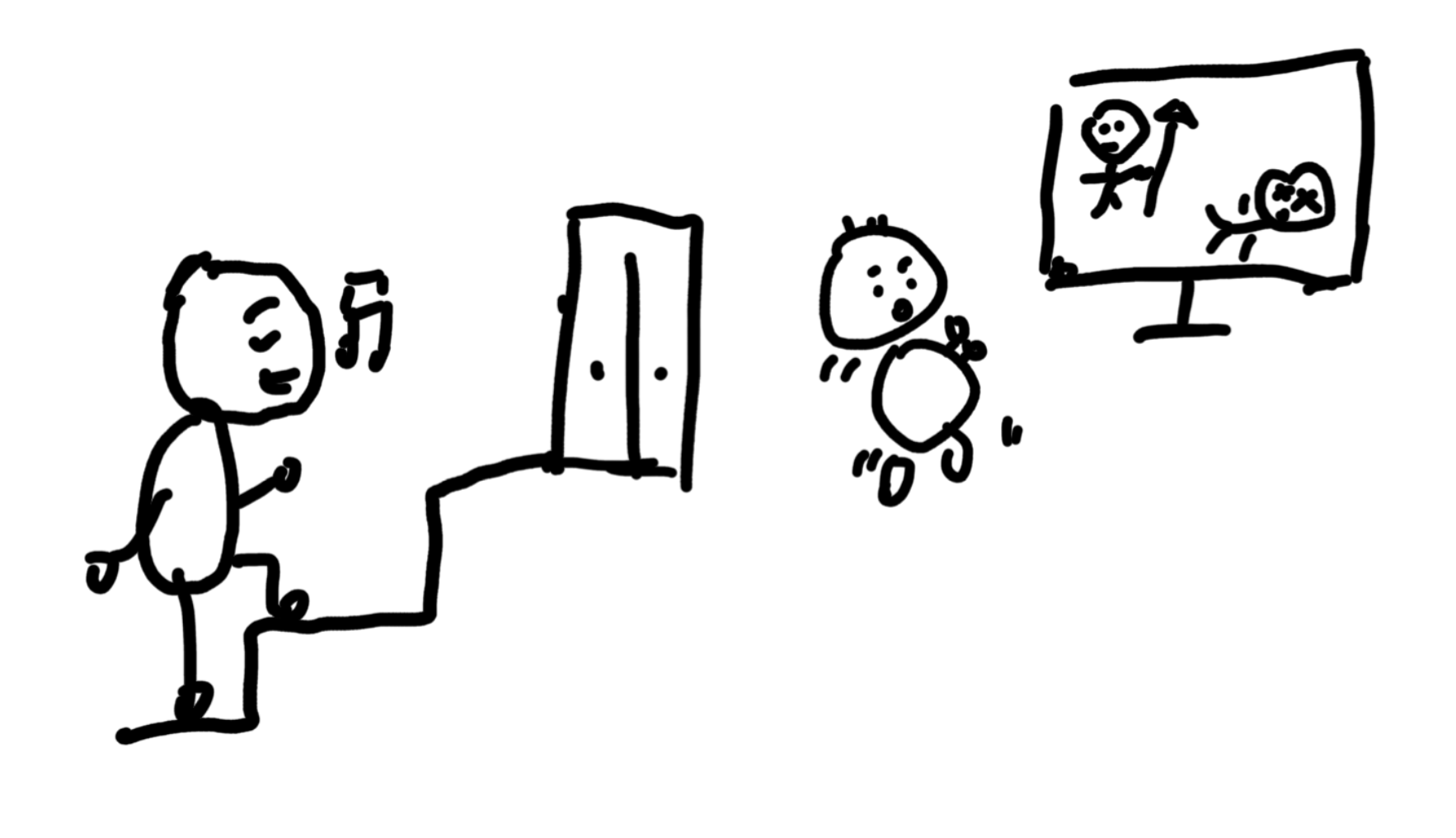
在我小的时候,经常趁父母出去上班不在家的空挡偷偷打开电视,看一些古侠剧(天龙八部、神雕侠侣…),里边的各种情节都十分吸引我。但是,我无时无刻不得不关注的就是门外的脚步声,那个时候只要二老回家,脚步声听的是清清楚楚,直接电视关机飞到小桌子前假装写作业一气呵成。没想到小小年纪便用到了我高贵的侧信道攻击(Side Channel Attack,SCA)技术!也就是说我并不需要看到他俩这个主体,通过上楼梯时所泄露出来的脚步声这一侧信息就可以判别是不是他们。
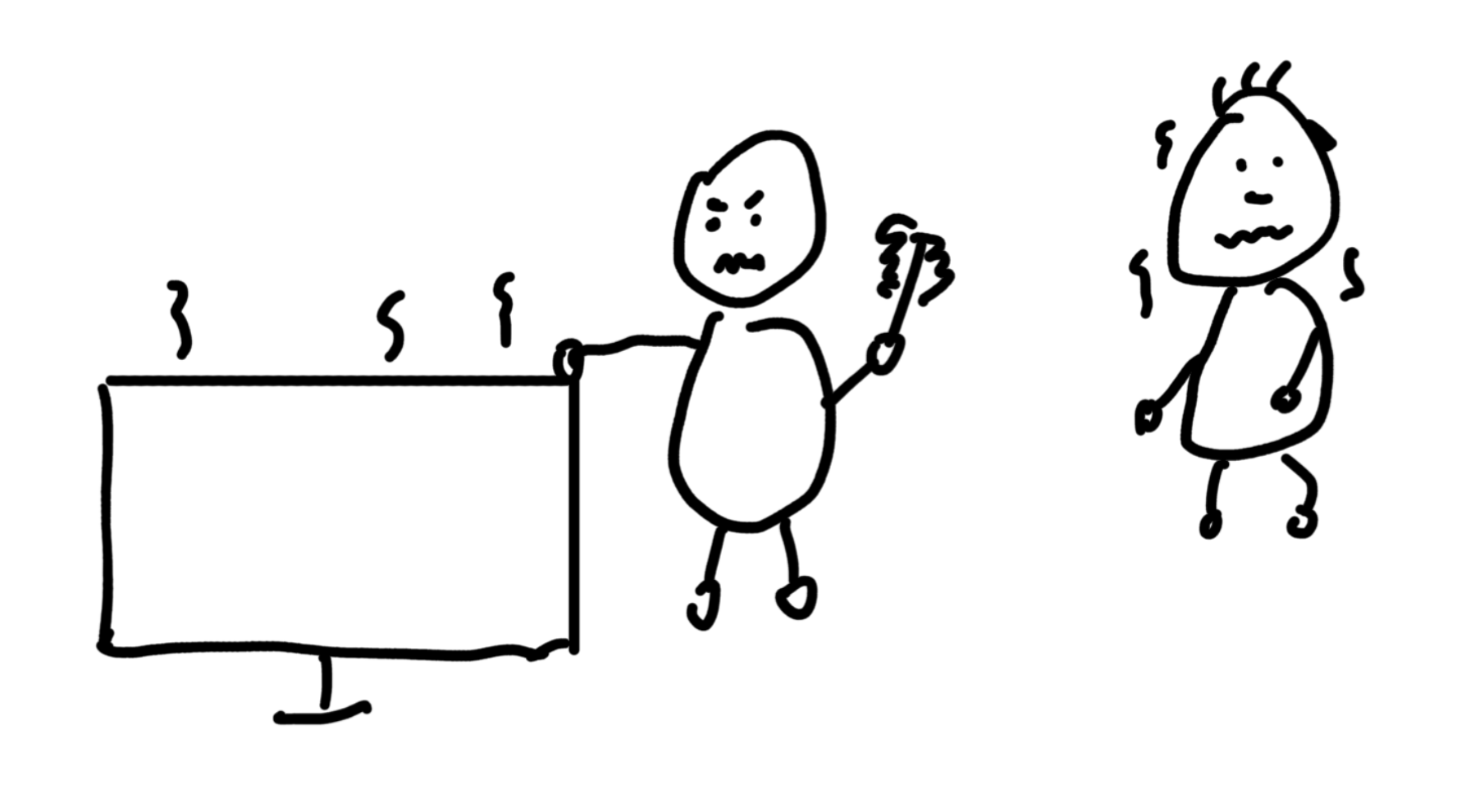
更令我没想到的是,后来他们也用到了侧信道分析!并不需要看到我在看电视,而是仅仅需要摸一下电视的大脑袋,通过电视机壳传出来的温度侧信息,去决定是否要对我发起物理攻击(不是。
在密码学领域中,侧信道攻击并不是直接针对的密码的算法层面,相较于其他理论上的分析攻击方法,该方法直接对设备加密时所泄漏的侧信息进行分析。例如在RSA密码算法中,当加密算法执行到求平方乘步骤时,指数位为0与为1所需要的执行耗时是不同的,攻击者可以通过观察执行泄露的时间侧信息来进行处理,进而恢复密钥。
侧信道攻击又分为建模类侧信道攻击(Profiling Side Channel Attack)以及非建模类侧信道攻击(Non-Profiling Side Channel Attack)。建模类侧信道攻击要求攻击者事先拥有一个与被攻击设备相同或相似的副本,通过采集在副本设备上执行密码算法所泄露出来的侧信息并加以分析建模。最后,将建好的模型用在被攻击设备上所泄漏的侧信息中,以完成关键信息的恢复,常见的攻击方法有模板攻击、随机模型攻击以及基于深度学习的侧信道攻击。非建模类侧信道攻击则直接对被攻击设备发起攻击,常见的攻击方法有SPA、DPA、CPA。
如何入门侧信道攻击
由于本人研究方向为侧信道攻击与深度学习的交叉学科(Deep Learning based Side Channel Attack,DLSCA),所以在学习相关知识的时候更偏向于深度学习建模的方法,大致分为兴趣点选择、数据预处理、特征工程、模型的训练、评估五部分[1],具体如图1所示。
以我个人经验,需要先搞懂的是你要建模的侧信息是什么,以下是在攻击时常用的数据集。每个数据集中都包含了大量的能量迹,每条能量迹又存在对应的时间样本点。之后的事情就是看论文做实验,下一部分有一些可以直接上手的基础实验,SCA_Box中的实验大家在做的时候如果有疑问可以直接在上边提Issue或Email联系我,我看到了都会回复。
需要复现的实验(有源码)
目前,DLSCA的论文有很多都给出了代码,大家有时间都要复现一下。我在下边贴出来论文以及对应代码链接,还有一些基础实验的复现代码,可以结合自己情况审代码以及实现。
基础实验:
- SCA_Box: https://github.com/i4mhmh/SCA_Box-Side-Channel-Attack-Tool-chest
- 信噪比:https://github.com/ileanabuhan/LeakageAssessment/blob/main/SNR-as-distingusher.ipynb
- 感知信息:https://github.com/ileanabuhan/LeakageAssessment/blob/main/What_is_PI.ipynb
论文及代码
- Study of Deep Learning Techniques for Side-Channel Analysis and Introduction to ASCAD Database
- Exploring Feature Selection Scenarios for Deep Learning-based Side-Channel Analysis
- Give Me 5 Minutes Attacking ASCAD with a Single Side-Channel Trace
- Revisiting a Methodology for Efficient CNN Architectures in Profiling Attacks
- The Need for Speed: A Fast Guessing Entropy Calculation for Deep Learning-Based SCA
- Label Correlation in Deep Learning-based Side-channel Analysis
- Weakly Profiling Side-channel Analysis
期刊
期刊 | 链接🔗 | 评级 |
---|---|---|
TCHES | https://tches.iacr.org/ | 顶刊⭐️⭐️⭐️⭐️+ |
JCEN 此生必投!!! | https://link.springer.com/journal/13389 | 中科院四区 JCR Q2 ⭐️⭐️⭐️ |
ePrint | https://eprint.iacr.org/ | 预印本(占坑) |
TIFS | https://ieeexplore.ieee.org/xpl/RecentIssue.jsp?punumber=10206 | 中科院一区 JCRQ1 ⭐️⭐️⭐️⭐️⭐️ |
Joc | ⭐️⭐️⭐️⭐️⭐️ |
会议
会议 | 链接 |
---|---|
ICCD | https://www.iccd-conf.com/Home.html |
参考文献
SoK: Deep Learning-based Physical Side-channel Analysis ↩︎